Robust progressive learning For GPR full waveform inversion
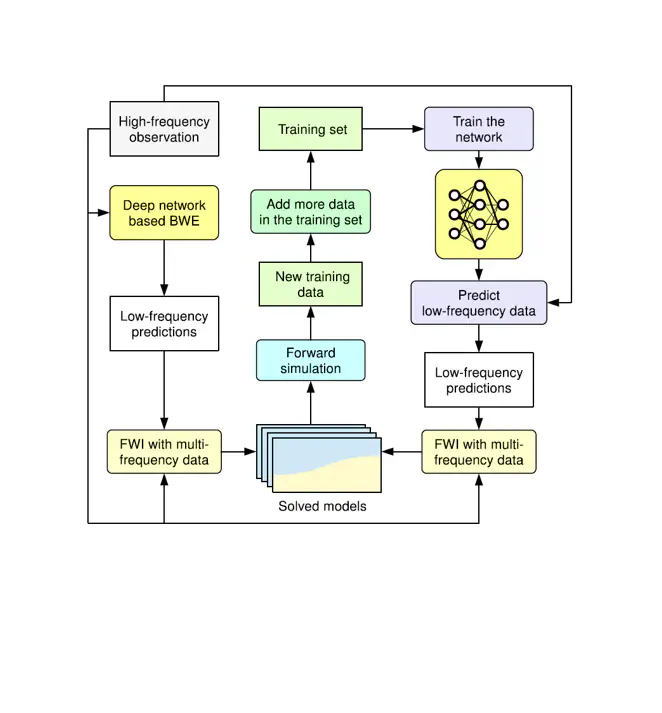
Figure Caption
Workflow of FWI using low-frequency data predicted by Robust Prossive Learning (RPL).
Research Summary
I participated in this research program led by Yuchen Jin from the Department of Electrical and Computer Engineering under the supervision of Dr. Wenyi Hu, Xuqing Wu, and , Prof. Jiefu Chen.
We proposed a robust progressive learning (RPL) method for the time-domain FWI. Although PL shows improvement on FWI, it requires repeatedly retraining the DNN with a small training dataset. There is no guarantee for the performance to be improved in each loop. The new RPL workflow utilizes an unsupervised learning method to reduce computational costs at the initialization stage. During the DNN training stage, we propose a self-regularized training scheme and use an augmented training dataset to improve the stability of the DNN model. The effectiveness of the proposed algorithm is verified by ISP and GPR inversion. The experiment results show that our proposed method successfully reconstructed models with the extrapolated low-frequency data and improved the imaging quality of FWI.